The Acceleration of AI and its Implications for Healthcare
As we step into 2025, the AI landscape is experiencing a significant leap in capabilities. Just months ago, the ARC-AGI benchmark – designed to measure AI’s ability to tackle novel problems like humans do – was considered an insurmountable challenge. Created by François Chollet in 2019, this benchmark had humbled even the most advanced models, with none breaking past the 55% mark. That was until December 2024, when OpenAI announced O3, a new “reasoning model” that exceeded expectations with an 87% score, marking a significant inflection point in AI capability.
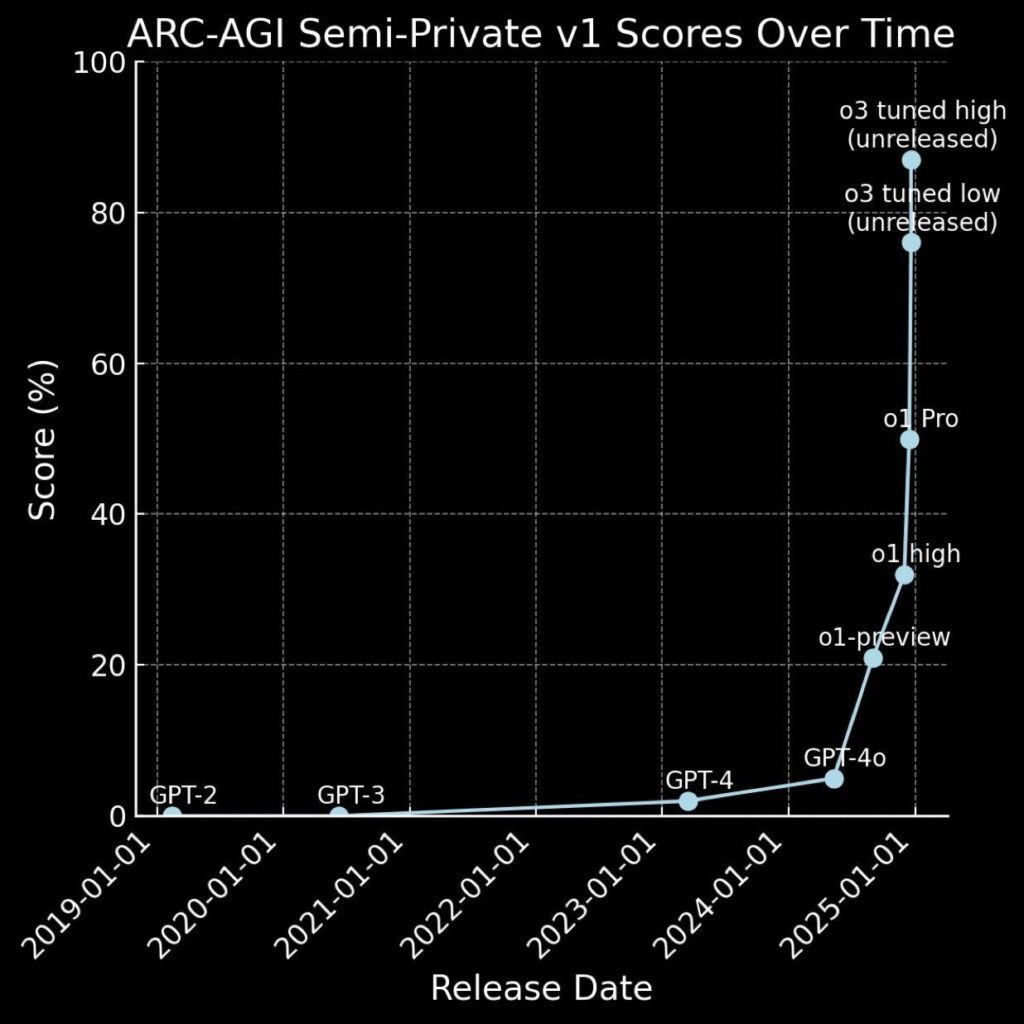
ARC-AGI Evaluation Scores for OpenAI Models Over Time (From 2019 - Present)
This doesn’t appear to be just another incremental improvement. The surge in performance spans across domains, from coding and computer science to more ‘hard’ sciences. Take the Graduate-Level Google-Proof Q&A (GPQA) test – a benchmark that includes hundreds of questions developed by experts across a variety of STEM fields. When PhDs attempt the questions, they reach a score of about 80% when answering questions in their field, and about 33% when answering questions outside their fields. In the second half of 2024, we saw the state-of-the-art on this benchmark go from the ~60% that Claude 3.5 sonnet scored, to ~80% for O1 and 87% (exceeding human experts) for the unreleased O3. More telling is that this progress isn’t limited to just one company or lab. Open-source models from labs like DeepSeek are rapidly catching up to frontier models at a fraction of the cost, democratizing access to advanced AI capabilities.
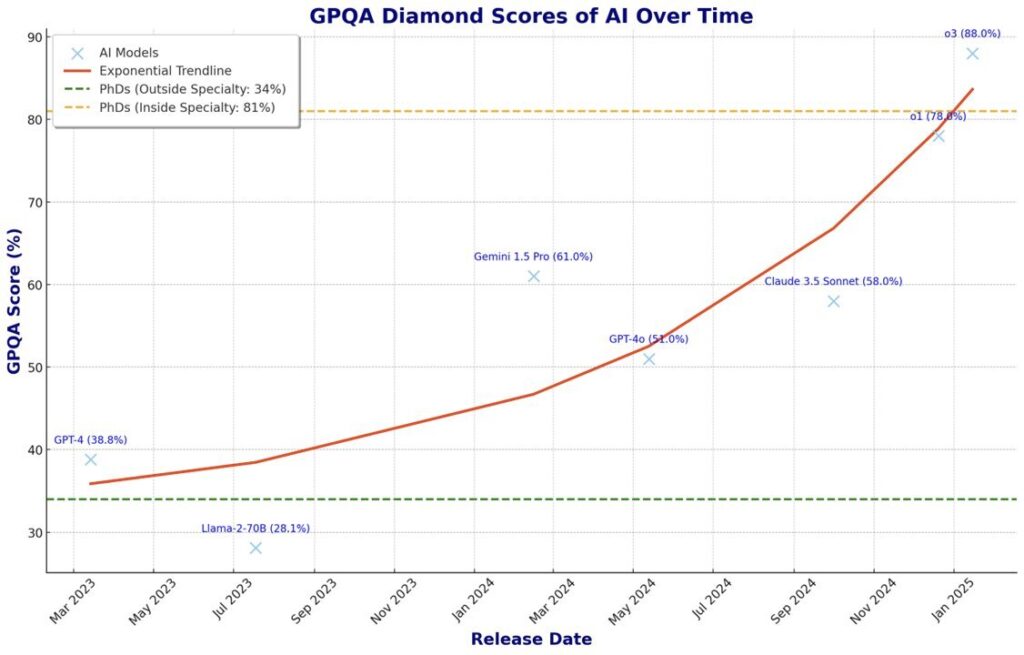
GPQA Scores for Frontier LLMs Over Time. The green line indicates the average score for PhDs who answered questions outside their field of expertise. The yellow line represents the average score for PhDs answering questions in their field of expertise.
But what does this mean for healthcare? A recent Harvard study provides an interesting perspective. Their evaluation of OpenAI’s O1-preview model against medical professionals on a new clinical reasoning benchmark yielded surprising results: the AI significantly outperformed not just dedicated clinical diagnostic tools, but also physicians – even when those physicians had access to AI assistance. On questions from the NEJM Clinicopathologic Conferences (CPCs) – a standard for the evaluation of differential diagnosis generators since the 1950s – O1-preview achieved 76% accuracy in differential diagnosis generation, nearly triple the performance of clinicians.
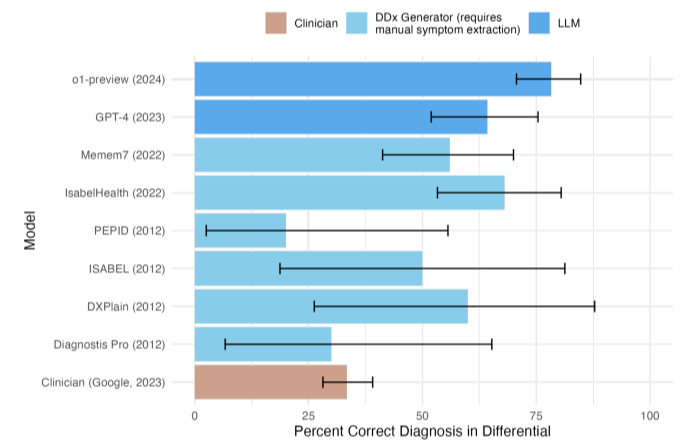
Barplot comparing the accuracy of including the correct diagnosis in the differential for differential diagnosis (DDx) generators, LLMs and clinicians on the NEJM CPCs.
The implications for neuromonitoring and clinical decision making could be significant. As a field that relies heavily on pattern recognition and complex data analysis, we’re uniquely positioned to benefit from these advances. However, important challenges remain, from ongoing concerns about model reliability and hallucinations to unresolved questions about training data and intellectual property rights. These challenges will need careful consideration as we move forward.
We’re witnessing what could be a transformative year for AI, particularly in healthcare. While the technology is advancing rapidly, successful integration will require thoughtful approaches to validation and implementation. As we navigate this evolving landscape, the key will be finding the right balance between embracing new capabilities and maintaining rigorous standards of care.