RESOURCES
PUBLICATIONS
YOUR RESEARCH RESOURCE
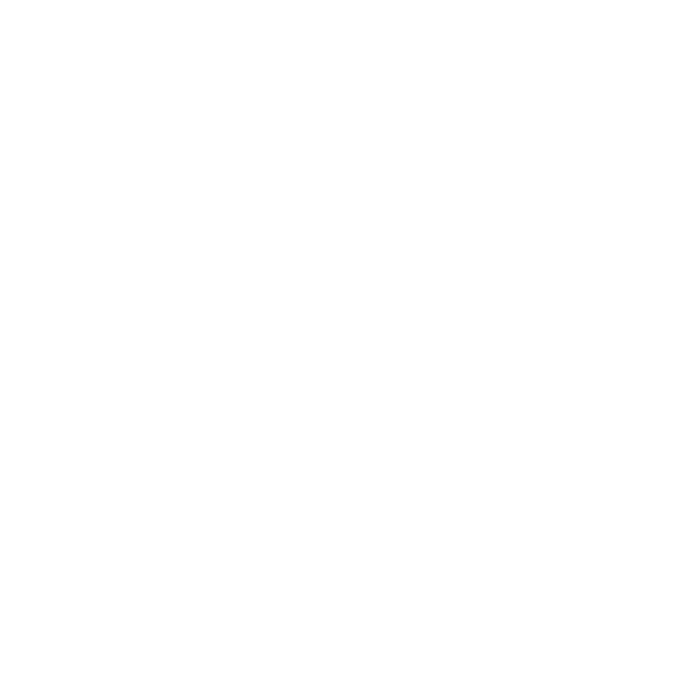
Moberg Analytics is authoring ground-breaking research and publications with some of the top neurocritical care institutions, physicians, nurses, and researchers.
Comparison of NIRS-Derived MAPopt Between Moberg Analytics and ICM+ in Pediatric Post-Cardiac Arrest Patients
Moore, J., Moyer, E.J., et al., Kirschen, M.
Moore, J., Moyer, E.J., et al., Kirschen, M.
Brain tissue oxygen saturation (StO₂) from near-infrared spectroscopy (NIRS) helps determine the optimal mean arterial pressure (MAPopt) for cerebral autoregulation (CA), but consistency across platforms remains unclear. This study compared MAPopt agreement between ICM+ and Moberg Analytics systems in 92 pediatric cardiac arrest patients, using single- and multi-window approaches with and without artifact reduction (AR). Single-window MAPopt availability was similar (48% vs. 48%, p=0.16), with a mean absolute difference of 1.0mmHg and 94% of differences within ±5mmHg. Multi-window analysis improved availability (ICM+: 76%, Moberg: 79%, p<0.001) but slightly reduced agreement (mean absolute difference: 2.5mmHg, 87% within ±5mmHg). AR further increased availability without affecting agreement. While MAPopt values were comparable, differences between platforms underscore the need for standardization to ensure reliable clinical use.
Predicting the Trajectory of Intracranial Pressure in Patients with Traumatic Brain Injury: Evaluation of a Foundation Model for Time Series
Van Leeuwen, F.D., Bhattacharyay, S., et al., Moberg, R.
Van Leeuwen, F.D., Bhattacharyay, S., et al., Moberg, R.
Patients with traumatic brain injury (TBI) often experience pathological increases in intracranial pressure (ICP), leading to intracranial hypertension (tIH), a common and serious complication. Early warning of an impending rise in ICP could potentially improve patient outcomes by enabling preemptive clinical intervention. However, the limited availability of patient data poses a challenge in developing reliable prediction models. In this study, we aim to determine whether foundation models, which leverage transfer learning, may offer a promising solution.
Using Physiological Biomarkers to Optimize Management of TBI in Austere Environments
Moberg, D., Moyer, E.J., et al., Jarema, D.
Moberg, D., Moyer, E.J., et al., Jarema, D.
Multimodal monitoring, which integrates data from multiple physiological sensors for individualized patient management, is increasingly used in civilian traumatic brain injury care. We hypothesized that this technology could be adapted for battlefield use with a noninvasive sensor suite and an AI-driven patient management guidance system. Collaborating with military medical personnel, we developed a hand-held system tailored to neurocritical care in combat settings. To optimize sensor selection, we introduced a Value-Burden Analysis, assessing both clinical utility and deployment feasibility, resulting in a weighted score based on the Role of Care. We evaluated 17 sensors and designed a modular guidance system incorporating interoperable components, a Knowledge Base, and AI-driven decision support aligned with Clinical Practice Guidelines for Traumatic Brain Injury in Prolonged Care. A prototype demonstrated feasibility, successfully simulating physiological inputs to match current guideline recommendations. This adaptable, modular system can integrate evolving sensors, resources, and clinical protocols, with ongoing validation through simulated scenarios.
Pitfalls and Possibilities of Using Root SedLine for Continuous Assessment of EEG Waveform-Based Metrics in Intensive Care Research
Bögli, S.Y., Cherchi, M.S., et al., Smielewski, P.
Bögli, S.Y., Cherchi, M.S., et al., Smielewski, P.
The Root SedLine device is utilized for continuous electroencephalography (cEEG)-based sedation monitoring in intensive care patients. cEEG traces can be collected for further processing and calculating additional metrics. However, depending on the device settings during acquisition, the traces may be affected by max/min value cropping or high digitization errors. Our goal was to systematically evaluate the impact of these distortions on the metrics used in clinical research within the field of neuromonitoring.
The Development of a TBI Navigator to Augment Management of Brain Injured Patients in Austere Environments
Moberg, R., Flesher, K., et al., Moyer, E.J.
Moberg, R., Flesher, K., et al., Moyer, E.J.
Future wars are expected to increase traumatic brain injuries (TBI), overwhelming battlefield medics. To help, we developed a TBI Navigator app, using guidelines from sources like the Brain Trauma Foundation and MACE2/MACE-T. This app creates a comprehensive medical record on a mobile phone, transforming guidelines into assessments and management steps. It triggers management recommendations based on patient conditions and is designed for use in resource-limited environments. Interoperable data structures and common terminology allow easy integration of new modules and devices. This app supports the 2023 DoD Data, Analytics, and AI Adoption Strategy, paving the way for commercialization.
Presented at SOMA 2024
Advancing InTBIR's Goals: A Platform for Large0Scale Physiological Data Analytics
Moyer, E.J., Maddux, C., et al., Moberg, R.
Moyer, E.J., Maddux, C., et al., Moberg, R.
The International Initiative for Traumatic Brain Injury Research (InTBIR) highlights the need for improved ICU monitoring technology for TBI management. To address this, we developed the Moberg Cloud Platform, a cloud-based system for uploading, reviewing, annotating, analyzing, and exporting high-resolution neurophysiology data from the neuro ICU. This platform is used in the BOOST3 trial and other TBI studies, including TRACK-TBI, in collaboration with Dr. Brandon Foreman at the University of Cincinnati. It was presented to the TRACK-TBI ICU working group in December 2023 and is being evaluated for the Curing Coma Campaign’s Coma Quality Improvement Program. Our platform’s features support InTBIR’s goals by providing centralized access to high-resolution TBI datasets. Future plans include implementing third-party algorithms, integrating with external databases like FITBIR, and validating real-time use in neuro ICUs. We are also developing a reimbursement strategy for multimodal neuromonitoring.
Presented at InTBIR 2024
Towards the Management of Ground Truth Consensus-Based Knowledge in Neurocritical Care
Moyer, E.J., Faang, X., et al., Moberg, D.
Moyer, E.J., Faang, X., et al., Moberg, D.
When information is isolated across literature publications, it can be difficult to see how it all fits together. This is especially true in neurocritical care. Through previous work, our group has extensively reviewed concepts related to harmonization. After exploring many concepts like ontology maps, reference dictionaries, etc., we have determined that a knowledge base is the optimal solution for this problem. This publication serves as preliminary work in an attempt to store, elevate, and connect ground truth knowledge in the field that drives the harmonization of concepts, ideas, and data. We aim to extend this work by incorporating this knowledge into CONNECT.
Presented at NCS 2023
Integration of High-Resolution Multimodal Neuromonitoring Data and Hospital EMR for Automated Near Real-Time Patient Care Reports
Maddux, C., Kirschen, M., et al., Moberg, D.
Maddux, C., Kirschen, M., et al., Moberg, D.
In a close collaboration with the Children’s Hospital of Philadelphia (CHOP), we have successfully been able to deliver neuromonitoring reports for pediatric patients at routine intervals. These reports combine artifact-reduced neurophysiology (ICP, ABP, HR, rSO2, etc.), computer trends (PRx, CPPopt, COx, MAPopt), continuous and non-continuous medication administration, summary metrics, clinical impressions, and a technical summary into a one- to two-page report. The report has been designed with the help of Dr. Matthew Kirschen. Our aim is to continue to deploy and validate this technology across hospitals all over the country.
Presented at NCS 2023
Methodology of Measuring the Precise Temporal-Spatial Behavior of Electrographic Seizures in Full Term Newborns with Arterio-Ischemic Strokes
Fung, F., Moyer, E.J., et al., Clancy, R.R.
Fung, F., Moyer, E.J., et al., Clancy, R.R.
Seizures following arterio-ischemic stroke in neonates are focal in nature but may spread to other areas of the brain, making them ideal to study the dynamics of seizure evolution in terms of duration and location. We developed a novel, reduced EEG montage and tested it against a cohort of 29 neonates to quantify the spatial and temporal behavior of electrographic seizures.
Presented at NCS 2023
Assessing the Clinical Value and Deployability of TBI Monitoring Technology for Prolonged Casualty Care
Gomba, M.A., Moyer, E.J., et al., Moberg, D.
Gomba, M.A., Moyer, E.J., et al., Moberg, D.
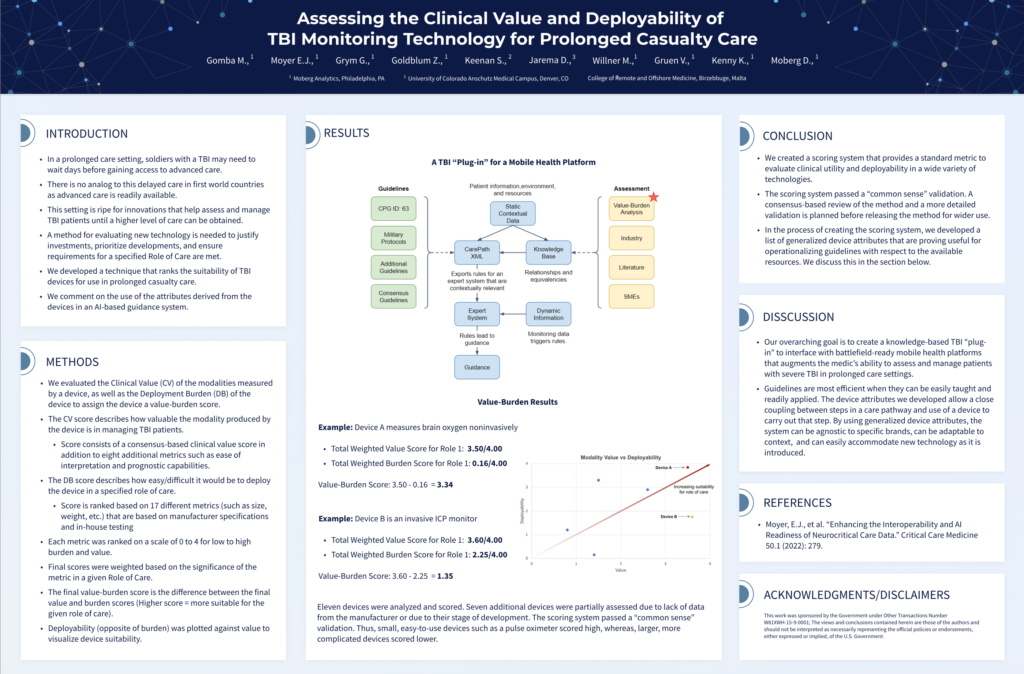
The content of a medic’s pack is incredibly important. If they want to add something in, they likely have to take something out. This is increasingly important in prolonged care scenarios where resources may be limited. Our poster highlights a method we developed in-house for evaluating devices and technology in terms of which are most optimal to use in these environments. Our method crosses the deployability burden of a device – how difficult it is to use in a certain environment – with the value of the measurement(s) it records. By crossing these two scores for over a dozen non-invasive sensors and devices, we were able to rank them in terms of how optimal they are for battlefield use.
Presented at SOMA 2023
Data Anonymization for Cloud-Based Storage of an Extensible Archive Format in Neurocritical Care
Moyer, E.J., Vo, Q.T., et al., Moberg, D.
Moyer, E.J., Vo, Q.T., et al., Moberg, D.
High-resolution data in neurocritical care is enormous, especially for patients that are monitored for days to weeks at a time. This type of data is ideal for machine learning applications, but certain barriers have traditionally thwarted this type of work. Data acquired from medical devices are often stored in proprietary company formats, making it difficult to use for research. Additionally, this data often contains PHI and cannot be moved outside of the hospital without an intermediate anonymization step. Our work highlights a solution to both of these problems. With the help of Dr. Eddy Amorim at the Zuckerberg San Francisco General Hospital, we successfully deployed software to anonymize and convert data from the Moberg CNS Monitor with the aim to use the hospital’s dataset for research.
Presented at NCS 2022
A Taxonomy for Defining Derived Metrics in Neurocritical Care for Machine Learning
Gruen, V., Kenny, K., et al., Moyer, E.J.
Gruen, V., Kenny, K., et al., Moyer, E.J.
Reproducibility is a huge problem across all disciplines of science. As the analyses and algorithms become more complex in neurocritical care, it will be more and more difficult for researchers to standardize their algorithms and effectively communicate them to others. We have a unique opportunity to solve this issue early on before it gets out of hand. This poster highlights preliminary work on a method for standardizing analytics using a pallet of interoperable, modular, and simple functions (standard math operations or reference to a publication describing an algorithm). Each metric produced by this method is computed with a metadata string that describes how the metric was derived.
Presented at NCS 2022
A Gap Analysis of Common Data Elements for Machine Learning in Neurocritical Care
Kenny, K., Gruen, V., et al., Moberg, D.
Kenny, K., Gruen, V., et al., Moberg, D.
Common Data Elements (CDEs) from the National Institute of Neurological Disorders and Stroke (NINDS) aim to standardize data annotations in research but lack key metadata for neurocritical care. This study conducted a gap analysis on CDEs related to traumatic brain injury (TBI) monitoring, assessing their relevance and modifying thresholds to align with clinical practice. By evaluating neurocritical care modalities, we identified gaps in data acquisition and annotations, refining the CDE database to enhance machine learning applications. Our findings highlight the need for expanded metadata to support nuanced multimodal data analysis. Future work includes integrating annotations into a relational Knowledge Base and creating a framework for continuous updates reflecting advancements in neurocritical care.
Presented at NCS 2022
Decision Support in Neurocritical Care Driven by Real-Time Physiology
Willner, M., Kenny, K., et al., Moberg, D.
Willner, M., Kenny, K., et al., Moberg, D.
Clinical decision support tools exist in neurocritical care, but none fully integrate real-time physiological data. We developed a proof-of-concept expert system that links neurological medications, monitoring modalities, and guideline-based thresholds. Using an ontology of ICU medications and physiological metrics, the system categorizes conditions and pre-conditions to advise clinical actions. Evaluated with a simulator and Moberg CNS monitor, it demonstrated feasibility in real-time decision support. Future work will expand the knowledge base to enhance neurophysiological monitoring and assist treatment decisions.
Presented at NCS 2022
CONNECT: A Platform for High-Resolution Multimodal Data Management for Clinical Trials of Brain Injured Patients
Moyer, E.J., Maddux, C., et al., Moberg, D.
Moyer, E.J., Maddux, C., et al., Moberg, D.
Live Presentation at INTS 2022
Harmonization of Physiological Data in Neurocritical Care: Challenges and a Path Forward
Moberg, R., Moyer, E.J., et al., Foreman, B.
Moberg, R., Moyer, E.J., et al., Foreman, B.
Continuous multimodal monitoring in neurocritical care provides valuable insights into the dynamics of the injured brain. Unfortunately, the “readiness” of this data for robust artificial intelligence (AI) and machine learning (ML) applications is low and presents a significant barrier for advancement. Harmonization standards and tools to implement those standards are key to overcoming existing barriers. Consensus in our professional community is essential for success.
Precision Care in Cardiac Arrest ICECAP (PRECICECAP) Study Protocol and Informatics Approach
Elmer, J., He, Z., [...], Hirsch, K.G., PRECICECAP Study Team
Elmer, J., He, Z., [...], Hirsch, K.G., PRECICECAP Study Team
The PRECICECAP study applies machine learning to multimodal, high-resolution data from out-of-hospital cardiac arrest patients to identify biomarker signatures predicting optimal therapeutic hypothermia duration and 90-day functional outcomes. Integrating data from the ICECAP trial, we collect detailed medication records, physiological waveforms, and imaging data. In collaboration with Moberg Analytics, we are developing a freely available software platform for standardized curation of ICU-acquired data, enabling efficient machine learning applications. Using autoencoder neural networks and supervised deep learning, we aim to refine patient-specific treatment strategies. PRECICECAP is actively enrolling and is expected to conclude in late 2025, contributing to personalized neurocritical care and broader hospital-based research applications.
A Novel Annotation Tools Using Multimodality Neuromonitoring Data Superimposed with Clinically-Relevant Events for Machine Learning
Moyer, E.J., Moberg, D., et al., Olson, D.
Moyer, E.J., Moberg, D., et al., Olson, D.
Multimodal neuromonitoring aids brain injury management, but lacks synchronized therapeutic action recording, limiting machine learning applications. We developed an annotation tool integrating multimodal data with clinical events for improved context. Collaborating with experts, we created an interactive visualization and annotation system, interfacing with existing data harmonization pipelines. A beta version is deployed in major brain injury trials. Future work includes real-time functionality, advanced analytics, and clinical validation.
Presented at NNS 2022
Semi-Automatic Pediatric Multimodal Neuromonitoring Reports
Moyer, E.J., Kuoch, E., et al., Moberg, D.
Moyer, E.J., Kuoch, E., et al., Moberg, D.
Multimodal neuromonitoring enhances brain assessment, but generating cEEG reports is time-consuming, especially for pediatric patients. This study develops a semi-automated system for EMR-ready neuro monitoring reports, integrating qualitative and quantitative neurometrics. Partnering with Phoenix Children’s Hospital, we automate data extraction and use a standardized web-based framework for customizable reports. Our prototype streamlines multimodal reporting, with future work focused on advanced analytics, visualization, and clinical validation.
Presented at PAS 2022
Enhancing the Interoperability and AI Readiness of Neurocritical Care Data
Moyer, E.J., Lawrence, S., et al., Moberg, D.
Moyer, E.J., Lawrence, S., et al., Moberg, D.
Artificial intelligence (AI) has the potential to revolutionize neurocritical care (NCC) by enabling precision management of brain injury patients. However, barriers such as data standardization, annotation, and device connectivity hinder its widespread adoption. With funding from the U.S. Department of Defense, we developed a standardized data infrastructure to make NCC data AI-ready. Our work focused on data integration, harmonization, standardized formats, and a user-friendly analytics dashboard. We established cloud connectivity for data uploads, developed an ontology for structured knowledge, standardized annotations, and converted data into HDF5 format with an API for access. A web-based dashboard was created for data visualization and interoperability. These tools have been successfully validated in clinical trials and AI-based seizure detection studies. Our efforts lay the groundwork for real-time, continuous precision management of brain-injured patients.
A Multimodal Monitoring Approach to Predicting Onset of Physiological Incidents Using Machine Learning
Moyer, E.J., Isozaki, I., Moberg, D.
Moyer, E.J., Isozaki, I., Moberg, D.
Traumatic brain injury (TBI) is a complex condition requiring continuous monitoring in the ICU. This study uses multimodal monitoring (MMM) and machine learning to predict physiological incidents – high intracranial pressure (ICP), out-of-range systolic arterial blood pressure (ABP), and out-of-range diastolic ABP – up to an hour before onset. Using data from 36 TRACK-TBI patients, we extracted 3,528 features from seven physiological modalities. We evaluated multiple classifiers, with random forest performing best, particularly for high ICP prediction. Future work includes expanding the cohort, assessing site-specific biases, and integrating additional modalities.
Presented at the IEEE Signal Processing in Medicine and Biology Symposium, December 2021
Using Contextual Data to Enhance Machine Learning in Traumatic Brain Injury
Goldblum, Z., Olson, D., et al., Moberg, D.
Goldblum, Z., Olson, D., et al., Moberg, D.
Contextual data is rarely incorporated into machine learning models for physiological event detection and prediction. In traumatic brain injury (TBI) patients, intracranial pressure (ICP) measurements are only valid when the external ventricular drain (EVD) stopcock is correctly positioned. We developed a stopcock position sensor using an inertial measurement unit (IMU) to record position data, transmitted via Bluetooth for time-synchronized annotation. Initial clinical review deemed the device functional, and four hours of continuous data demonstrated accurate synchronization. Future work includes clinical evaluation at UT Southwestern and expanding contextual data integration for ICU and battlefield applications.
Presented at NCS 2021 & MHSRS 2021
Development and Validation of an Open-Source Optimum Cerebral Perfusion Pressure Tool to Guide Precision Care in Every Traumatic Brain Injury
Foreman, B., Fei, L., et al., Moberg, D.
Foreman, B., Fei, L., et al., Moberg, D.
Live presentation at NCS 2021
The Accuracy of Coma Prediction Improves During the First 3 Days of Neurocritical Care and is Influenced by Severity and Persistence of Neurologic Deterioration: Results from the CHoRUS Workgroup
Sharma, G., Nooney, S., et al., Rosenthal, E.S.
Sharma, G., Nooney, S., et al., Rosenthal, E.S.
Live presentation at NCS 2021
Extracting Meaning from Neurocritical Care Annotations Requires a Brain Injury-Specific Natural Language Processing Vocabulary: Progress from the MIND Workgroup
Sharma, G., Olson, D.M., et al., Rosenthal, E.S.
Sharma, G., Olson, D.M., et al., Rosenthal, E.S.
Live presentation at NCS 2021
A Cloud-Based Platform for Data Management in Traumatic Brain Injury Clinical Trials (CONNECT): Progress from the MIND Workgroup
Stover, J., Maddux, C., et al., Moberg, D.
Stover, J., Maddux, C., et al., Moberg, D.
Traumatic brain injury (TBI) trials require comprehensive, high-resolution data management, but past efforts have struggled with synchronization, metadata tracking, and centralized storage. The CONNECT platform addresses these gaps by providing a cloud-based, de-identified data repository with real-time management and analysis tools. Data are harmonized into HDF5 format, synchronized with electronic medical records (EMRs), and stored on IBM Cloud with AWS S3 access. CONNECT supports secure data uploads, open-source API access, and quality assurance workflows. Successfully tested in BOOST-3 and TRACK-TBI, the platform will be deployed in PRECICECAP and ELECTRO-BOOST. Future work includes broader validation, austere environment applications, and automated trial data review.
Presented at MHSRS 2021
A Robust Data Archive Format for Traumatic Brain Injury Physiology and Machine Learning: Progress from the MIND Workgroup
Habboush, D., Rosenthal, E.S., et al., Moberg, D.
Habboush, D., Rosenthal, E.S., et al., Moberg, D.
Providing military medical care in austere environments requires a multimodal approach to optimize TBI patient management. The MIND group has developed a common data format using HDF5, enabling high-resolution physiological data storage, metadata integration, and real-time analysis for patient state assessment and trajectory prediction. 257 patient files (742GB) from TRACK-TBI were converted into HDF5, achieving a 62.8% reduction in file size via Gzip and scale-offset compression. Successfully implemented in IBM Cloud, this format is currently in use for BOOST-3 and TRACK-TBI trials. Future work will expand device compatibility and data sources to enhance neurocritical care research and clinical applications.
Presented at MHSRS 2021 & NCS 2021
The Severity and Persistence of Neurological Deterioration are Associated with Clinical Outcome: Progress from the MIND Workgroup
Sharma, G., Nooney, S., et al., Rosenthal, E.S.
Sharma, G., Nooney, S., et al., Rosenthal, E.S.
Live Presentation at MHSRS 2021
Development and Validation of an Open-Source, Online Optimum Cerebral Perfusion Pressure Tool to Guide Precision are in Severe Traumatic Brain Injury: Progress for the MIND Workgroup
Foreman, B., Li, F., et al., Moberg, D.
Foreman, B., Li, F., et al., Moberg, D.
Live Presentation at MHSRS 2021
MIND Collaborative Effort to Developing Meaning from Annotated Data During Continuous Multimodal Monitoring
Olson, D., Sharma, G., et al., Rosenthal, E.S.
Olson, D., Sharma, G., et al., Rosenthal, E.S.
Live Presentation at the International Neuroscience Nursing Research Symposium 2021
Challenges and Opportunities in Multimodal Monitoring and Data Analytics in Traumatic Brain Injury
Foreman, B., Lissak, I.A., et al., Rosenthal, E.S.
Foreman, B., Lissak, I.A., et al., Rosenthal, E.S.
Increasingly sophisticated systems for monitoring the brain have led to an increase in the use of multimodality monitoring (MMM) to detect secondary brain injuries before irreversible damage occurs after brain trauma. This review examines the challenges and opportunities associated with MMM in this population. Locally and internationally, the use of MMM varies. Practical challenges include difficulties with data acquisition, curation, and harmonization with other data sources limiting collaboration. However, efforts toward integration of MMM data, advancements in data science, and the availability of cloud-based infrastructures are now affording the opportunity for MMM to advance the care of patients with brain trauma. MMM provides data to guide the precision management of patients with traumatic brain injury in real time. While challenges exist, there are exciting opportunities for MMM to live up to this promise and to drive new insights into the physiology of the brain and beyond.
Comparison of NIRS-Derived MAPopt Between Moberg Analytics and ICM+ in Pediatric Post-Cardiac Arrest Patients
Moore, J., Moyer, E.J., et al., Kirschen, M.
Moore, J., Moyer, E.J., et al., Kirschen, M.
Brain tissue oxygen saturation (StO₂) from near-infrared spectroscopy (NIRS) helps determine the optimal mean arterial pressure (MAPopt) for cerebral autoregulation (CA), but consistency across platforms remains unclear. This study compared MAPopt agreement between ICM+ and Moberg Analytics systems in 92 pediatric cardiac arrest patients, using single- and multi-window approaches with and without artifact reduction (AR). Single-window MAPopt availability was similar (48% vs. 48%, p=0.16), with a mean absolute difference of 1.0mmHg and 94% of differences within ±5mmHg. Multi-window analysis improved availability (ICM+: 76%, Moberg: 79%, p<0.001) but slightly reduced agreement (mean absolute difference: 2.5mmHg, 87% within ±5mmHg). AR further increased availability without affecting agreement. While MAPopt values were comparable, differences between platforms underscore the need for standardization to ensure reliable clinical use.
Predicting the Trajectory of Intracranial Pressure in Patients with Traumatic Brain Injury: Evaluation of a Foundation Model for Time Series
Van Leeuwen, F.D., Bhattacharyay, S., et al., Moberg, R.
Van Leeuwen, F.D., Bhattacharyay, S., et al., Moberg, R.
Patients with traumatic brain injury (TBI) often experience pathological increases in intracranial pressure (ICP), leading to intracranial hypertension (tIH), a common and serious complication. Early warning of an impending rise in ICP could potentially improve patient outcomes by enabling preemptive clinical intervention. However, the limited availability of patient data poses a challenge in developing reliable prediction models. In this study, we aim to determine whether foundation models, which leverage transfer learning, may offer a promising solution.
Using Physiological Biomarkers to Optimize Management of TBI in Austere Environments
Moberg, D., Moyer, E.J., et al., Jarema, D.
Moberg, D., Moyer, E.J., et al., Jarema, D.
Multimodal monitoring, which integrates data from multiple physiological sensors for individualized patient management, is increasingly used in civilian traumatic brain injury care. We hypothesized that this technology could be adapted for battlefield use with a noninvasive sensor suite and an AI-driven patient management guidance system. Collaborating with military medical personnel, we developed a hand-held system tailored to neurocritical care in combat settings. To optimize sensor selection, we introduced a Value-Burden Analysis, assessing both clinical utility and deployment feasibility, resulting in a weighted score based on the Role of Care. We evaluated 17 sensors and designed a modular guidance system incorporating interoperable components, a Knowledge Base, and AI-driven decision support aligned with Clinical Practice Guidelines for Traumatic Brain Injury in Prolonged Care. A prototype demonstrated feasibility, successfully simulating physiological inputs to match current guideline recommendations. This adaptable, modular system can integrate evolving sensors, resources, and clinical protocols, with ongoing validation through simulated scenarios.
Pitfalls and Possibilities of Using Root SedLine for Continuous Assessment of EEG Waveform-Based Metrics in Intensive Care Research
Bögli, S.Y., Cherchi, M.S., et al., Smielewski, P.
Bögli, S.Y., Cherchi, M.S., et al., Smielewski, P.
The Root SedLine device is utilized for continuous electroencephalography (cEEG)-based sedation monitoring in intensive care patients. cEEG traces can be collected for further processing and calculating additional metrics. However, depending on the device settings during acquisition, the traces may be affected by max/min value cropping or high digitization errors. Our goal was to systematically evaluate the impact of these distortions on the metrics used in clinical research within the field of neuromonitoring.
The Development of a TBI Navigator to Augment Management of Brain Injured Patients in Austere Environments
Moberg, R., Flesher, K., et al., Moyer, E.J.
Moberg, R., Flesher, K., et al., Moyer, E.J.
Future wars are expected to increase traumatic brain injuries (TBI), overwhelming battlefield medics. To help, we developed a TBI Navigator app, using guidelines from sources like the Brain Trauma Foundation and MACE2/MACE-T. This app creates a comprehensive medical record on a mobile phone, transforming guidelines into assessments and management steps. It triggers management recommendations based on patient conditions and is designed for use in resource-limited environments. Interoperable data structures and common terminology allow easy integration of new modules and devices. This app supports the 2023 DoD Data, Analytics, and AI Adoption Strategy, paving the way for commercialization.
Presented at SOMA 2024
Advancing InTBIR's Goals: A Platform for Large0Scale Physiological Data Analytics
Moyer, E.J., Maddux, C., et al., Moberg, R.
Moyer, E.J., Maddux, C., et al., Moberg, R.
The International Initiative for Traumatic Brain Injury Research (InTBIR) highlights the need for improved ICU monitoring technology for TBI management. To address this, we developed the Moberg Cloud Platform, a cloud-based system for uploading, reviewing, annotating, analyzing, and exporting high-resolution neurophysiology data from the neuro ICU. This platform is used in the BOOST3 trial and other TBI studies, including TRACK-TBI, in collaboration with Dr. Brandon Foreman at the University of Cincinnati. It was presented to the TRACK-TBI ICU working group in December 2023 and is being evaluated for the Curing Coma Campaign’s Coma Quality Improvement Program. Our platform’s features support InTBIR’s goals by providing centralized access to high-resolution TBI datasets. Future plans include implementing third-party algorithms, integrating with external databases like FITBIR, and validating real-time use in neuro ICUs. We are also developing a reimbursement strategy for multimodal neuromonitoring.
Presented at InTBIR 2024
Towards the Management of Ground Truth Consensus-Based Knowledge in Neurocritical Care
Moyer, E.J., Faang, X., et al., Moberg, D.
Moyer, E.J., Faang, X., et al., Moberg, D.
When information is isolated across literature publications, it can be difficult to see how it all fits together. This is especially true in neurocritical care. Through previous work, our group has extensively reviewed concepts related to harmonization. After exploring many concepts like ontology maps, reference dictionaries, etc., we have determined that a knowledge base is the optimal solution for this problem. This publication serves as preliminary work in an attempt to store, elevate, and connect ground truth knowledge in the field that drives the harmonization of concepts, ideas, and data. We aim to extend this work by incorporating this knowledge into CONNECT.
Presented at NCS 2023
Integration of High-Resolution Multimodal Neuromonitoring Data and Hospital EMR for Automated Near Real-Time Patient Care Reports
Maddux, C., Kirschen, M., et al., Moberg, D.
Maddux, C., Kirschen, M., et al., Moberg, D.
In a close collaboration with the Children’s Hospital of Philadelphia (CHOP), we have successfully been able to deliver neuromonitoring reports for pediatric patients at routine intervals. These reports combine artifact-reduced neurophysiology (ICP, ABP, HR, rSO2, etc.), computer trends (PRx, CPPopt, COx, MAPopt), continuous and non-continuous medication administration, summary metrics, clinical impressions, and a technical summary into a one- to two-page report. The report has been designed with the help of Dr. Matthew Kirschen. Our aim is to continue to deploy and validate this technology across hospitals all over the country.
Presented at NCS 2023
Methodology of Measuring the Precise Temporal-Spatial Behavior of Electrographic Seizures in Full Term Newborns with Arterio-Ischemic Strokes
Fung, F., Moyer, E.J., et al., Clancy, R.R.
Fung, F., Moyer, E.J., et al., Clancy, R.R.
Seizures following arterio-ischemic stroke in neonates are focal in nature but may spread to other areas of the brain, making them ideal to study the dynamics of seizure evolution in terms of duration and location. We developed a novel, reduced EEG montage and tested it against a cohort of 29 neonates to quantify the spatial and temporal behavior of electrographic seizures.
Presented at NCS 2023
Assessing the Clinical Value and Deployability of TBI Monitoring Technology for Prolonged Casualty Care
Gomba, M.A., Moyer, E.J., et al., Moberg, D.
Gomba, M.A., Moyer, E.J., et al., Moberg, D.
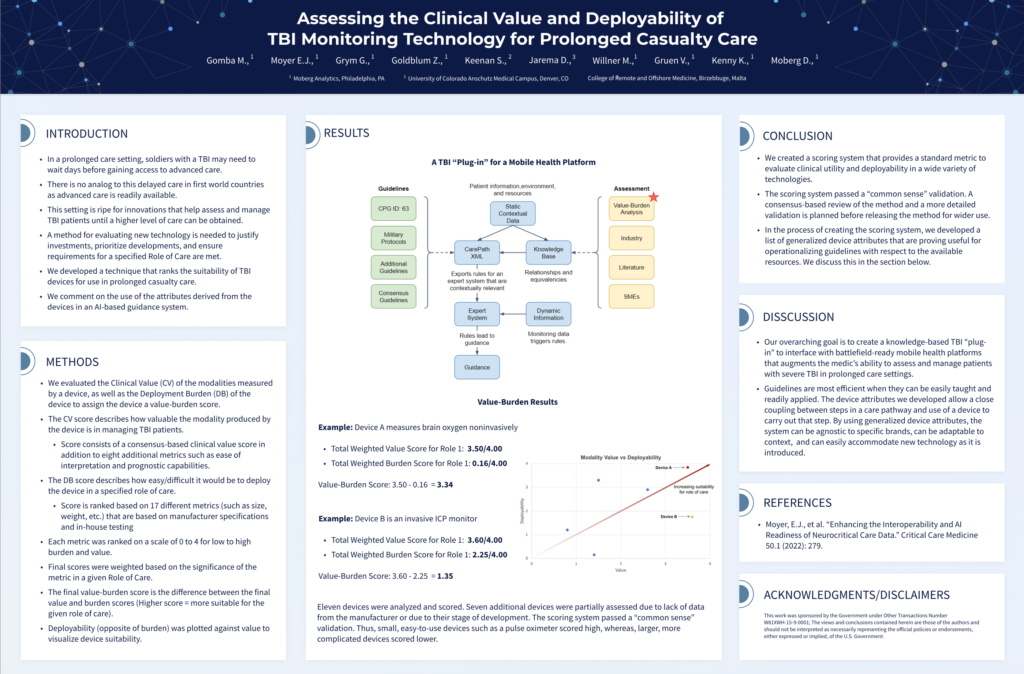
The content of a medic’s pack is incredibly important. If they want to add something in, they likely have to take something out. This is increasingly important in prolonged care scenarios where resources may be limited. Our poster highlights a method we developed in-house for evaluating devices and technology in terms of which are most optimal to use in these environments. Our method crosses the deployability burden of a device – how difficult it is to use in a certain environment – with the value of the measurement(s) it records. By crossing these two scores for over a dozen non-invasive sensors and devices, we were able to rank them in terms of how optimal they are for battlefield use.
Presented at SOMA 2023
Data Anonymization for Cloud-Based Storage of an Extensible Archive Format in Neurocritical Care
Moyer, E.J., Vo, Q.T., et al., Moberg, D.
Moyer, E.J., Vo, Q.T., et al., Moberg, D.
High-resolution data in neurocritical care is enormous, especially for patients that are monitored for days to weeks at a time. This type of data is ideal for machine learning applications, but certain barriers have traditionally thwarted this type of work. Data acquired from medical devices are often stored in proprietary company formats, making it difficult to use for research. Additionally, this data often contains PHI and cannot be moved outside of the hospital without an intermediate anonymization step. Our work highlights a solution to both of these problems. With the help of Dr. Eddy Amorim at the Zuckerberg San Francisco General Hospital, we successfully deployed software to anonymize and convert data from the Moberg CNS Monitor with the aim to use the hospital’s dataset for research.
Presented at NCS 2022
A Taxonomy for Defining Derived Metrics in Neurocritical Care for Machine Learning
Gruen, V., Kenny, K., et al., Moyer, E.J.
Gruen, V., Kenny, K., et al., Moyer, E.J.
Reproducibility is a huge problem across all disciplines of science. As the analyses and algorithms become more complex in neurocritical care, it will be more and more difficult for researchers to standardize their algorithms and effectively communicate them to others. We have a unique opportunity to solve this issue early on before it gets out of hand. This poster highlights preliminary work on a method for standardizing analytics using a pallet of interoperable, modular, and simple functions (standard math operations or reference to a publication describing an algorithm). Each metric produced by this method is computed with a metadata string that describes how the metric was derived.
Presented at NCS 2022
A Gap Analysis of Common Data Elements for Machine Learning in Neurocritical Care
Kenny, K., Gruen, V., et al., Moberg, D.
Kenny, K., Gruen, V., et al., Moberg, D.
Common Data Elements (CDEs) from the National Institute of Neurological Disorders and Stroke (NINDS) aim to standardize data annotations in research but lack key metadata for neurocritical care. This study conducted a gap analysis on CDEs related to traumatic brain injury (TBI) monitoring, assessing their relevance and modifying thresholds to align with clinical practice. By evaluating neurocritical care modalities, we identified gaps in data acquisition and annotations, refining the CDE database to enhance machine learning applications. Our findings highlight the need for expanded metadata to support nuanced multimodal data analysis. Future work includes integrating annotations into a relational Knowledge Base and creating a framework for continuous updates reflecting advancements in neurocritical care.
Presented at NCS 2022
Decision Support in Neurocritical Care Driven by Real-Time Physiology
Willner, M., Kenny, K., et al., Moberg, D.
Willner, M., Kenny, K., et al., Moberg, D.
Clinical decision support tools exist in neurocritical care, but none fully integrate real-time physiological data. We developed a proof-of-concept expert system that links neurological medications, monitoring modalities, and guideline-based thresholds. Using an ontology of ICU medications and physiological metrics, the system categorizes conditions and pre-conditions to advise clinical actions. Evaluated with a simulator and Moberg CNS monitor, it demonstrated feasibility in real-time decision support. Future work will expand the knowledge base to enhance neurophysiological monitoring and assist treatment decisions.
Presented at NCS 2022
CONNECT: A Platform for High-Resolution Multimodal Data Management for Clinical Trials of Brain Injured Patients
Moyer, E.J., Maddux, C., et al., Moberg, D.
Moyer, E.J., Maddux, C., et al., Moberg, D.
Live Presentation at INTS 2022
Harmonization of Physiological Data in Neurocritical Care: Challenges and a Path Forward
Moberg, R., Moyer, E.J., et al., Foreman, B.
Moberg, R., Moyer, E.J., et al., Foreman, B.
Continuous multimodal monitoring in neurocritical care provides valuable insights into the dynamics of the injured brain. Unfortunately, the “readiness” of this data for robust artificial intelligence (AI) and machine learning (ML) applications is low and presents a significant barrier for advancement. Harmonization standards and tools to implement those standards are key to overcoming existing barriers. Consensus in our professional community is essential for success.
Precision Care in Cardiac Arrest ICECAP (PRECICECAP) Study Protocol and Informatics Approach
Elmer, J., He, Z., [...], Hirsch, K.G., PRECICECAP Study Team
Elmer, J., He, Z., [...], Hirsch, K.G., PRECICECAP Study Team
The PRECICECAP study applies machine learning to multimodal, high-resolution data from out-of-hospital cardiac arrest patients to identify biomarker signatures predicting optimal therapeutic hypothermia duration and 90-day functional outcomes. Integrating data from the ICECAP trial, we collect detailed medication records, physiological waveforms, and imaging data. In collaboration with Moberg Analytics, we are developing a freely available software platform for standardized curation of ICU-acquired data, enabling efficient machine learning applications. Using autoencoder neural networks and supervised deep learning, we aim to refine patient-specific treatment strategies. PRECICECAP is actively enrolling and is expected to conclude in late 2025, contributing to personalized neurocritical care and broader hospital-based research applications.
A Novel Annotation Tools Using Multimodality Neuromonitoring Data Superimposed with Clinically-Relevant Events for Machine Learning
Moyer, E.J., Moberg, D., et al., Olson, D.
Moyer, E.J., Moberg, D., et al., Olson, D.
Multimodal neuromonitoring aids brain injury management, but lacks synchronized therapeutic action recording, limiting machine learning applications. We developed an annotation tool integrating multimodal data with clinical events for improved context. Collaborating with experts, we created an interactive visualization and annotation system, interfacing with existing data harmonization pipelines. A beta version is deployed in major brain injury trials. Future work includes real-time functionality, advanced analytics, and clinical validation.
Presented at NNS 2022
Semi-Automatic Pediatric Multimodal Neuromonitoring Reports
Moyer, E.J., Kuoch, E., et al., Moberg, D.
Moyer, E.J., Kuoch, E., et al., Moberg, D.
Multimodal neuromonitoring enhances brain assessment, but generating cEEG reports is time-consuming, especially for pediatric patients. This study develops a semi-automated system for EMR-ready neuro monitoring reports, integrating qualitative and quantitative neurometrics. Partnering with Phoenix Children’s Hospital, we automate data extraction and use a standardized web-based framework for customizable reports. Our prototype streamlines multimodal reporting, with future work focused on advanced analytics, visualization, and clinical validation.
Presented at PAS 2022
Enhancing the Interoperability and AI Readiness of Neurocritical Care Data
Moyer, E.J., Lawrence, S., et al., Moberg, D.
Moyer, E.J., Lawrence, S., et al., Moberg, D.
Artificial intelligence (AI) has the potential to revolutionize neurocritical care (NCC) by enabling precision management of brain injury patients. However, barriers such as data standardization, annotation, and device connectivity hinder its widespread adoption. With funding from the U.S. Department of Defense, we developed a standardized data infrastructure to make NCC data AI-ready. Our work focused on data integration, harmonization, standardized formats, and a user-friendly analytics dashboard. We established cloud connectivity for data uploads, developed an ontology for structured knowledge, standardized annotations, and converted data into HDF5 format with an API for access. A web-based dashboard was created for data visualization and interoperability. These tools have been successfully validated in clinical trials and AI-based seizure detection studies. Our efforts lay the groundwork for real-time, continuous precision management of brain-injured patients.
A Multimodal Monitoring Approach to Predicting Onset of Physiological Incidents Using Machine Learning
Moyer, E.J., Isozaki, I., Moberg, D.
Moyer, E.J., Isozaki, I., Moberg, D.
Traumatic brain injury (TBI) is a complex condition requiring continuous monitoring in the ICU. This study uses multimodal monitoring (MMM) and machine learning to predict physiological incidents – high intracranial pressure (ICP), out-of-range systolic arterial blood pressure (ABP), and out-of-range diastolic ABP – up to an hour before onset. Using data from 36 TRACK-TBI patients, we extracted 3,528 features from seven physiological modalities. We evaluated multiple classifiers, with random forest performing best, particularly for high ICP prediction. Future work includes expanding the cohort, assessing site-specific biases, and integrating additional modalities.
Presented at the IEEE Signal Processing in Medicine and Biology Symposium, December 2021
Using Contextual Data to Enhance Machine Learning in Traumatic Brain Injury
Goldblum, Z., Olson, D., et al., Moberg, D.
Goldblum, Z., Olson, D., et al., Moberg, D.
Contextual data is rarely incorporated into machine learning models for physiological event detection and prediction. In traumatic brain injury (TBI) patients, intracranial pressure (ICP) measurements are only valid when the external ventricular drain (EVD) stopcock is correctly positioned. We developed a stopcock position sensor using an inertial measurement unit (IMU) to record position data, transmitted via Bluetooth for time-synchronized annotation. Initial clinical review deemed the device functional, and four hours of continuous data demonstrated accurate synchronization. Future work includes clinical evaluation at UT Southwestern and expanding contextual data integration for ICU and battlefield applications.
Presented at NCS 2021 & MHSRS 2021
Development and Validation of an Open-Source Optimum Cerebral Perfusion Pressure Tool to Guide Precision Care in Every Traumatic Brain Injury
Foreman, B., Fei, L., et al., Moberg, D.
Foreman, B., Fei, L., et al., Moberg, D.
Live presentation at NCS 2021
The Accuracy of Coma Prediction Improves During the First 3 Days of Neurocritical Care and is Influenced by Severity and Persistence of Neurologic Deterioration: Results from the CHoRUS Workgroup
Sharma, G., Nooney, S., et al., Rosenthal, E.S.
Sharma, G., Nooney, S., et al., Rosenthal, E.S.
Live presentation at NCS 2021
Extracting Meaning from Neurocritical Care Annotations Requires a Brain Injury-Specific Natural Language Processing Vocabulary: Progress from the MIND Workgroup
Sharma, G., Olson, D.M., et al., Rosenthal, E.S.
Sharma, G., Olson, D.M., et al., Rosenthal, E.S.
Live presentation at NCS 2021
A Cloud-Based Platform for Data Management in Traumatic Brain Injury Clinical Trials (CONNECT): Progress from the MIND Workgroup
Stover, J., Maddux, C., et al., Moberg, D.
Stover, J., Maddux, C., et al., Moberg, D.
Traumatic brain injury (TBI) trials require comprehensive, high-resolution data management, but past efforts have struggled with synchronization, metadata tracking, and centralized storage. The CONNECT platform addresses these gaps by providing a cloud-based, de-identified data repository with real-time management and analysis tools. Data are harmonized into HDF5 format, synchronized with electronic medical records (EMRs), and stored on IBM Cloud with AWS S3 access. CONNECT supports secure data uploads, open-source API access, and quality assurance workflows. Successfully tested in BOOST-3 and TRACK-TBI, the platform will be deployed in PRECICECAP and ELECTRO-BOOST. Future work includes broader validation, austere environment applications, and automated trial data review.
Presented at MHSRS 2021
A Robust Data Archive Format for Traumatic Brain Injury Physiology and Machine Learning: Progress from the MIND Workgroup
Habboush, D., Rosenthal, E.S., et al., Moberg, D.
Habboush, D., Rosenthal, E.S., et al., Moberg, D.
Providing military medical care in austere environments requires a multimodal approach to optimize TBI patient management. The MIND group has developed a common data format using HDF5, enabling high-resolution physiological data storage, metadata integration, and real-time analysis for patient state assessment and trajectory prediction. 257 patient files (742GB) from TRACK-TBI were converted into HDF5, achieving a 62.8% reduction in file size via Gzip and scale-offset compression. Successfully implemented in IBM Cloud, this format is currently in use for BOOST-3 and TRACK-TBI trials. Future work will expand device compatibility and data sources to enhance neurocritical care research and clinical applications.
Presented at MHSRS 2021 & NCS 2021
The Severity and Persistence of Neurological Deterioration are Associated with Clinical Outcome: Progress from the MIND Workgroup
Sharma, G., Nooney, S., et al., Rosenthal, E.S.
Sharma, G., Nooney, S., et al., Rosenthal, E.S.
Live Presentation at MHSRS 2021
Development and Validation of an Open-Source, Online Optimum Cerebral Perfusion Pressure Tool to Guide Precision are in Severe Traumatic Brain Injury: Progress for the MIND Workgroup
Foreman, B., Li, F., et al., Moberg, D.
Foreman, B., Li, F., et al., Moberg, D.
Live Presentation at MHSRS 2021
MIND Collaborative Effort to Developing Meaning from Annotated Data During Continuous Multimodal Monitoring
Olson, D., Sharma, G., et al., Rosenthal, E.S.
Olson, D., Sharma, G., et al., Rosenthal, E.S.
Live Presentation at the International Neuroscience Nursing Research Symposium 2021
Challenges and Opportunities in Multimodal Monitoring and Data Analytics in Traumatic Brain Injury
Foreman, B., Lissak, I.A., et al., Rosenthal, E.S.
Foreman, B., Lissak, I.A., et al., Rosenthal, E.S.
Increasingly sophisticated systems for monitoring the brain have led to an increase in the use of multimodality monitoring (MMM) to detect secondary brain injuries before irreversible damage occurs after brain trauma. This review examines the challenges and opportunities associated with MMM in this population. Locally and internationally, the use of MMM varies. Practical challenges include difficulties with data acquisition, curation, and harmonization with other data sources limiting collaboration. However, efforts toward integration of MMM data, advancements in data science, and the availability of cloud-based infrastructures are now affording the opportunity for MMM to advance the care of patients with brain trauma. MMM provides data to guide the precision management of patients with traumatic brain injury in real time. While challenges exist, there are exciting opportunities for MMM to live up to this promise and to drive new insights into the physiology of the brain and beyond.