AI/ML: Only the Hard Problems Remain… For Now
We’ve watched AI/ML “grow up” and progress through increasingly difficult problems for nearly two decades. In its toddler years, it rode its tricycle around the kitchen and recommended books and movies for us. Pretty soon the training wheels were off and it was buzzing around the neighborhood.
We let it into the medical field and it helped us avoid readmissions and was able to give us early warnings on sepsis. Pretty cool stuff. It also flopped, sometimes spectacularly. Our own Chief Technologist was at IBM during its ambitious “moon shot” to provide real-time, bedside cancer treatment recommendations at MD Anderson. As moon shots go, the project ended more like Apollo One than Apollo Eleven, but someone had to go first and IBM could afford big, expensive “learning experiences.”
Seven years later, AI/ML seems to have been given the keys to the car. It’s not yet an adult, but it’s being put in some very adult situations. It’s showing promise on some very hard problems.
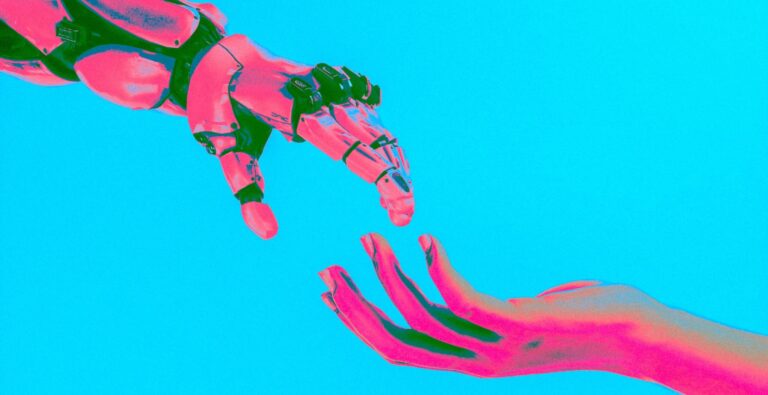
Efforts like CHoRUS, clinical trials like BOOST-3, and other new partnerships are allowing us to build the large, diverse datasets and ensure the model fairness and generalizability needed to attack these problems. We’ve established data sharing partnerships with Penn Medicine, UCSF, University of Cincinnati, Children’s Hospital of Philadelphia, and Drexel University to help push the use of AI/ML into TBI. Additionally, technologies like Federated Learning are allowing us to build ML-based models across institutions without sharing the data.

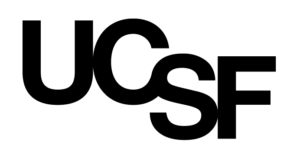



Just like these hard problems, hard issues are the last ones to be faced. We’ve solved most of the technical issues of AI/ML, but big non-technical issues remain, things like explainability and interpretability. Understanding the reasoning behind AI-assisted decisions and the ability to interpret those decisions is crucial. Without it, the use of AI/ML in clinical care will not be accepted by healthcare professionals.
It seems we have hit the peak of the generative AI hype cycle. Frankly, we welcome the change as we enter this next phase. AI/ML will grow into a true “adult” as we set it against our hardest TBI-related problems. Ensuring it is a well-behaved and fair adult will be our biggest challenge and most important responsibility.

In an effort to foster adoption of AI/ML in TBI, we will be hosting a webinar series this Fall designed to help clinicians learn how to get more from their neurocritical care data. Each session will focus on a particular real-world case study from us or from your colleagues. We will walk participants through the steps to re-create the analytics that answer the neurocritical care question presented. The sessions are offered free of charge. You can register here.