ChatGPT’s Matrix of Misinformation
Last week, the Guardian published an article highlighting a significant error found in ChatGPT. A Guardian journalist was approached by a researcher interested in reading more about an article cited by ChatGPT that was missing from their website. After involving other members from their organization including the legal department, their team determined that this article was never published and the chatbot simply generated it out of thin air. This happened on more than one occasion with other articles presented to the Guardian. The most troubling part of all of this is that the fake articles looked and sounded like something the Guardian would have published in the past.
Given the immense popularity of ChatGPT, this situation poses ethical questions about the reliability of statements coming from generative AI. As machine learning enthusiasts, we already know that there are inherent limitations to these models. In fact, there is a saying in the AI community that “all models are wrong, but some are useful.” When AI is deployed, it is sometimes difficult to determine when the scales tip and “wrong” outweighs “useful.”
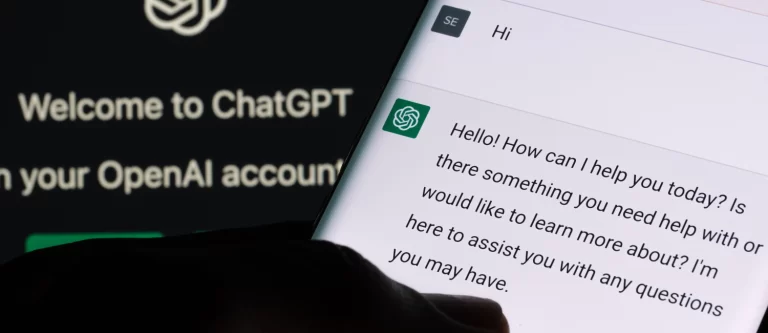
What is fake? What is real? How do you define real? Similar questions were posed in the 1999 film, The Matrix. Although you and Morpheous, the leader of the Matrix resistance in the movie, would likely have different answers, you two would agree that one single source of information leads to a deceptive reality. Afterall, if no one questioned the missing Guardian article, what would have stopped us from thinking that it existed? Neo, the movie’s protagonist, was fortunate to choose whether he would spend the rest of his life questioning reality. I am afraid that with the release of these chatbots, this choice has already been made for us.
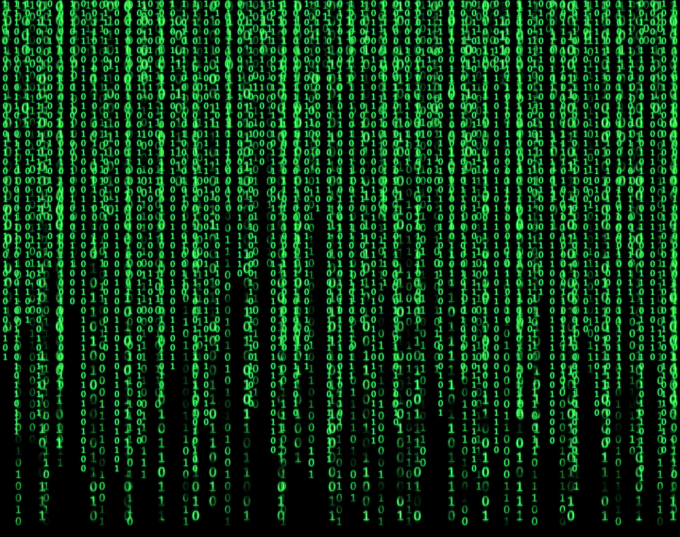
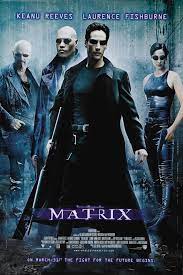
The future is less eerie for the neurocritical care field. Luckily, the medical field adopts technology at a much slower pace than most industries, allowing us to learn from their mistakes. Expert systems and, specifically, clinical decision support predate these advanced chatbots by over 70 years. These models do something that ChatGPT cannot: rationally explain decisions.
Instead of generating text to explain whether you should take the blue or red pill based on your personality profile (as ChatGPT would), expert systems will point to specific known rules decided by subject matter experts (and not fake Guardian articles). Building these systems require far less empirical data compared to what is required to train chatbots; however, they do require clear cut rules that may or may not be self-evident in the real world.
But then again, what is real?